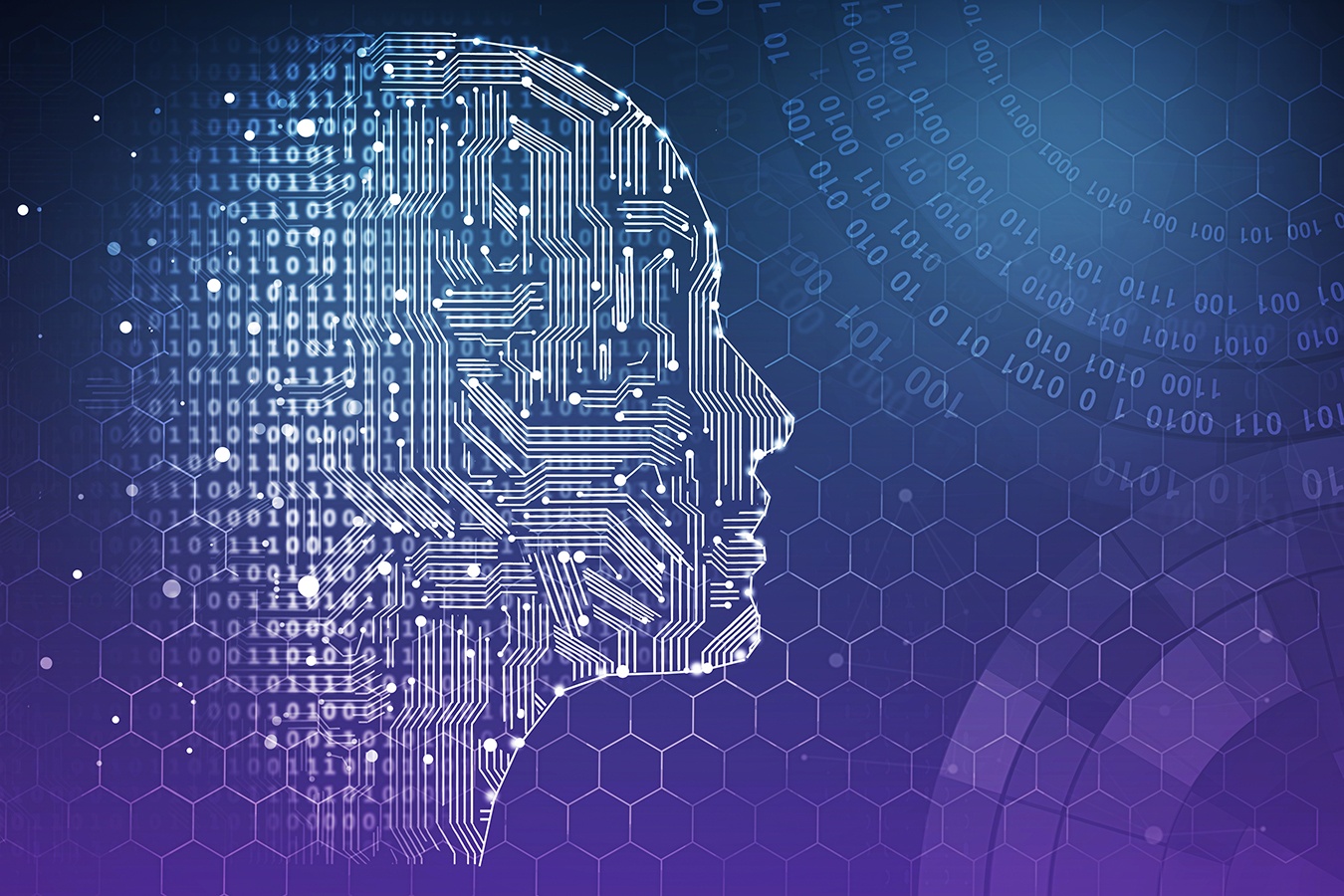
AI and Machine Learning: A Primer
We’ve been hearing about the potential impacts Artificial Intelligence (AI) could have on the legal profession for several years now. Naysayers warn of waves of legal administration job losses and that IBM’s Watson could supplant lawyers. Proponents laud AI’s ability to transform the legal industry to a model of effectiveness and profitability never seen before. Neither side provides clear and direct evidence of how these things might actually come to pass. The AI discussion outside of legal operates on the same basic premises, but at a macro level. Elon Musk and Stephen Hawking warn us that robots are going to take over the world, Facebook and Google tell us the technology will help us to save the world. In the midst of all the hype, the fact is that there is a lot of confusion around what AI is, how it works, and what the technology can actually do right now.
We aim to separate hype from reality in the conversation about AI in legal and how it could impact the profession at large. Not only is there a lot of confusion about how AI can be used in legal, there is a lot of confusion about the technology itself and the terms used to describe it. Therefore, we think it’s useful to start with exploring the distinctions between the two terms that get thrown around most often, AI and machine learning.
While AI and machine learning are inextricably linked, they’re not interchangeable. AI is essentially the overarching discipline in Computer Science that deals with building machines capable of intelligent behavior. AI can be broken down into two types, the moonshot type is “General” AI, or AI that exhibits all the same characteristics as human intelligence. General AI—a machine replication of humanity—isn’t really possible. Yet.
However, what today’s AI technology can do very well—and is getting better at all the time—is “Applied” or “Narrow” AI. Applied AI applications are ones that can do specific tasks just as well—or better, as is increasingly the case—than human beings. Think of Facebook’s facial recognition feature, for example, that can recognize your friends in a photo, or the self-parking feature in your car. All examples of Applied AI in action, each exhibit some approximation of human intelligence and reasoning. That intelligence and reasoning comes via machine learning.
Machine learning teaches computers to solve problems, rather than programming them to do a particular set of tasks. At its most basic, machine learning uses algorithms to break down data, learn from it, and then make a decision or prediction about something in the world. The theory behind machine learning is to program a computer to think like a human being and then give it access to enormous amounts of data—like the Internet—from which it can learn.
But how exactly does a machine learn? Essentially through a system of probability—based on data provided to the system, it makes statements or predictions with a degree of certainty. Then, when you tell the system whether it is right or wrong, it modifies the approach it takes and “learns” to make the right decisions, statements, and predictions more effectively, adjusting continuously through a constant feedback loop. Of course, as the network is “learning” it comes up with a lot of incorrect answers. But once it has seen hundreds of thousands or even millions of bits of data, the probability weightings can be tuned so precisely that it gets the answer right practically every time.
Machine learning is behind Netflix’s recommendations, learning from your viewing history and whether you give a show a thumbs up or a thumbs down. Systems like Google Play and Spotify use machine learning to determine what music you might like based on your listening history or whether you tell the system you feel like listening to something upbeat. Machine learning enables the system to recognize whether a piece of music will likely make listeners feel happy and will serve up suggestions accordingly. It can even teach a system to make music, such as the work being turned out by composer and scientist David Cope’s EMMY (short for Experiments in Musical Intelligence).
Anyone with a Gmail account will have noticed the “Smart Reply” feature that suggests responses to emails based on predictions driven by machine learning. And the system is clearly onto something—according to Google, 10 percent of all replies sent with Gmail were initiated using the Smart Reply feature. Because information about the use of Smart Replies is also fed back into the system, Smart Reply will also learn to make better, more accurate suggestions in the future.
Suggestions for movie night or music for your workout are one thing, but when we begin to think about the broader implications of applications like Smart Reply to something like process automation, we can see how machine learning could impact legal work in a beneficial way. The latest, biggest step forward in AI has been the evolution of machine learning into Deep Learning. Via a computer system called a Neural Network, AI systems can now classify information the same way a human brain does, but with enormous volumes of data—Big Data—the human brain could never manage on its own.
Legal is ruled by massive amounts of data and much of the costs associated with legal operations are focused on the consumption of that data effectively and efficiently. Deep Learning is what is driving AI forward and what might lead to AI solutions that could genuinely impact legal operations. As AI continues to develop, driven by advances in machine learning, the potential for valuable AI technology solutions in legal seems like more of a realistic possibility.